The relationship between mind and matter is perhaps the deepest intellectual challenge facing humanity. It links to wide ranges of science, philosophy and engineering, and has been infamously resistant to solution. In recent times, Artificial Intelligence (AI) has become a central theme in addressing this challenge, offering several insights that are both scientifically interesting and practically useful.
In the past decade, the field of Deep Neural Networks (DNNs) has brought renewed energy and focus to AI, through a series of remarkable breakthroughs in fields as diverse as speech recognition, board games and self-driving cars. In these and other applications, DNN systems have reached previously unknown levels of accuracy, making human-level performance a distinct possibility and thus suggesting novel insights on the mind-matter problem.
The successes of DNN systems have inspired much research into better algorithms, novel applications and a better understanding of DNNs. The MUST group is involved in all these aspects of DNN research. For example, we use DNNs to handle poor quality audio in speech and speaker recognition systems better, to probe the processes at play during solar flare eruptions, and even to optimise the design of sailplane wing shapes with some of our industry partners. We balance these applications with theoretical work focused on understanding and characterising generalisation in the context of deep learning. Irrespective of an extremely active research community studying these techniques, surprisingly many open questions remain.
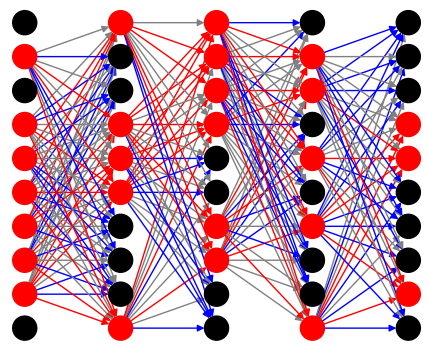
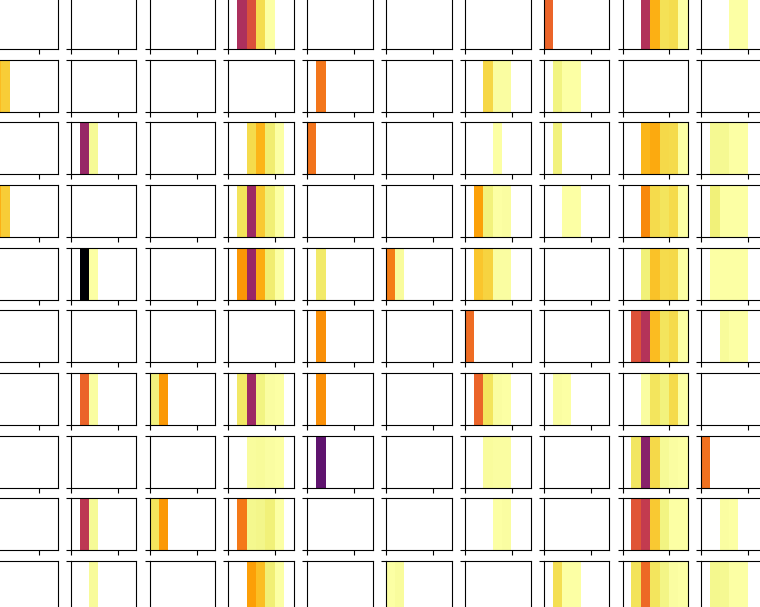
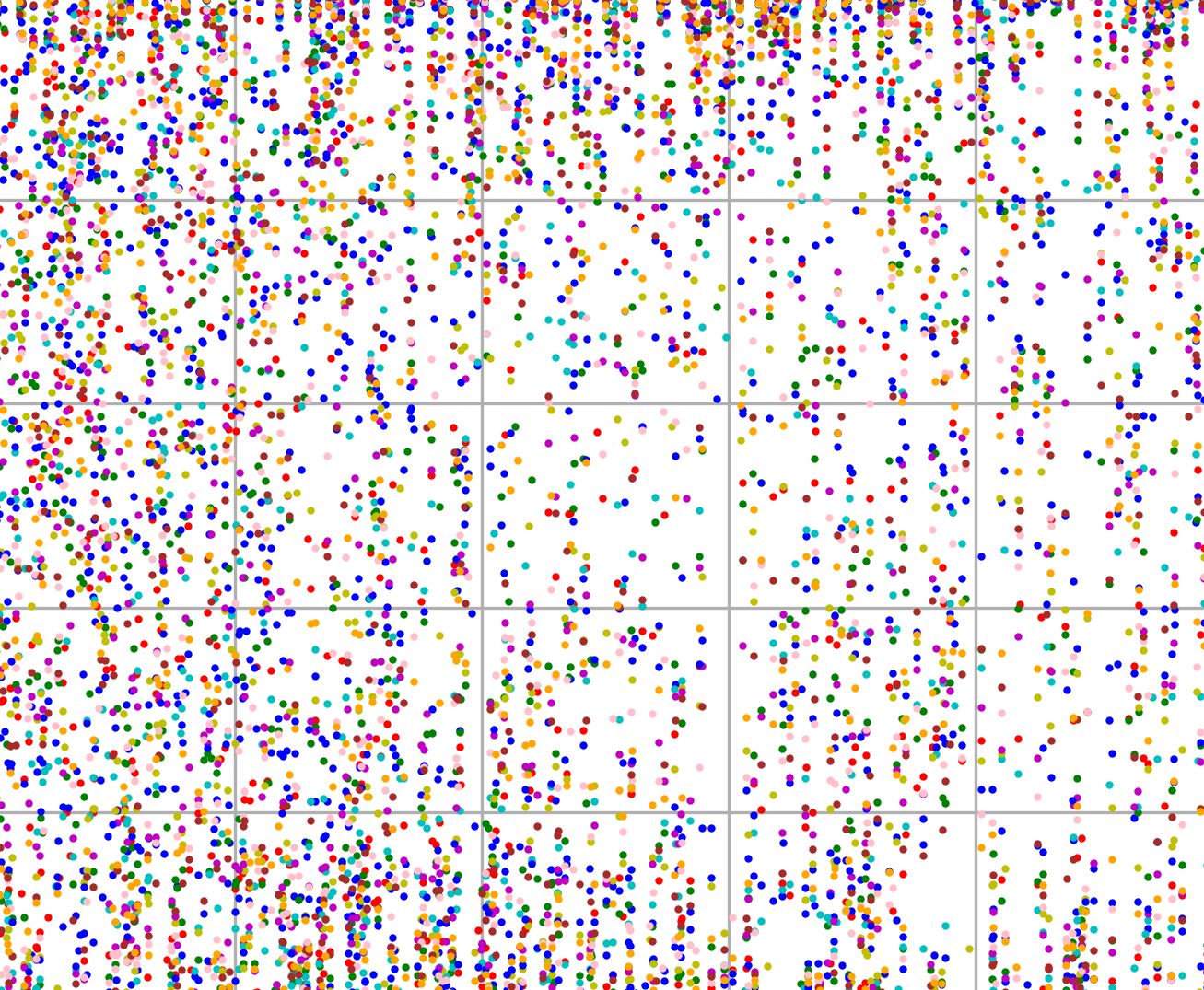
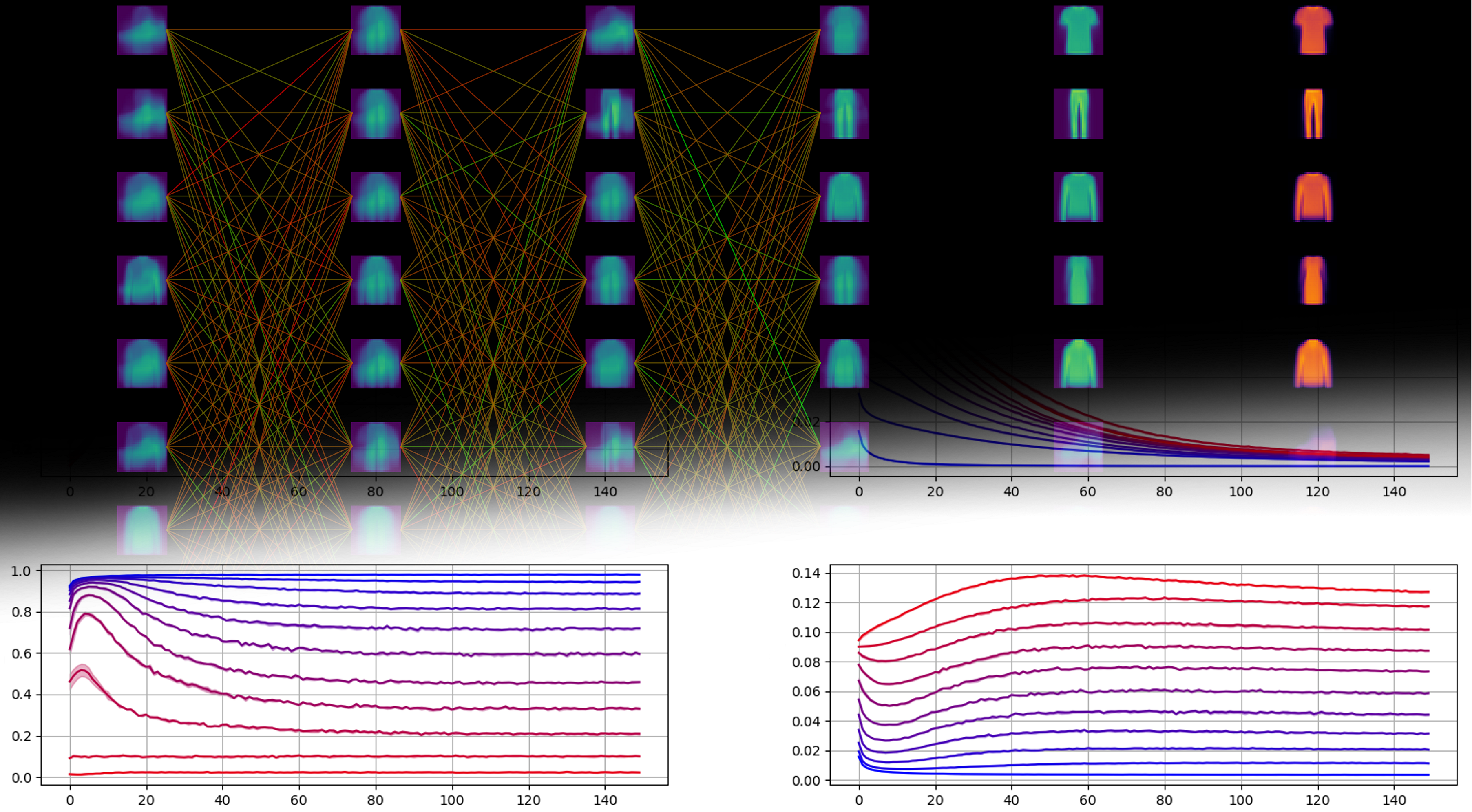
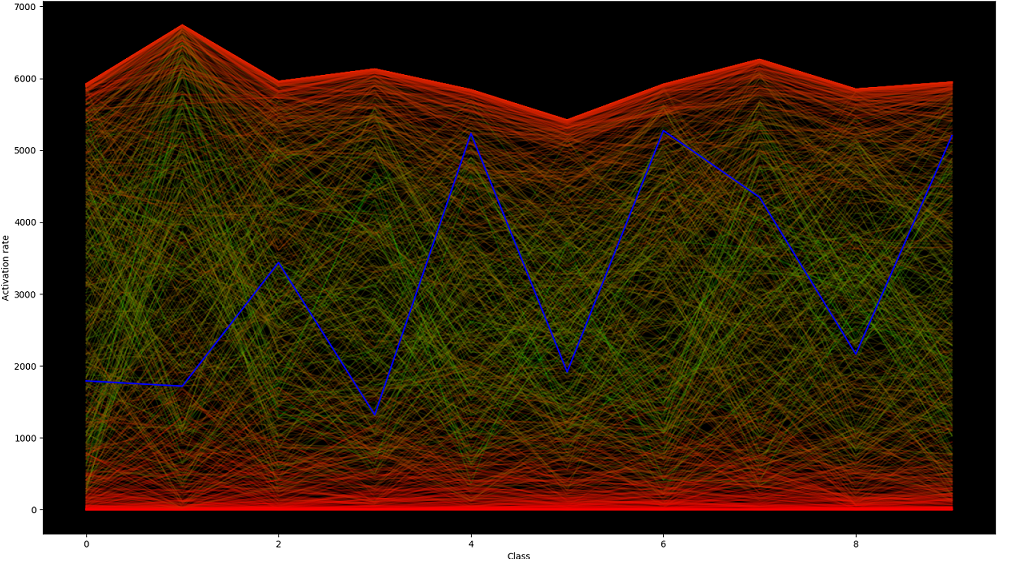
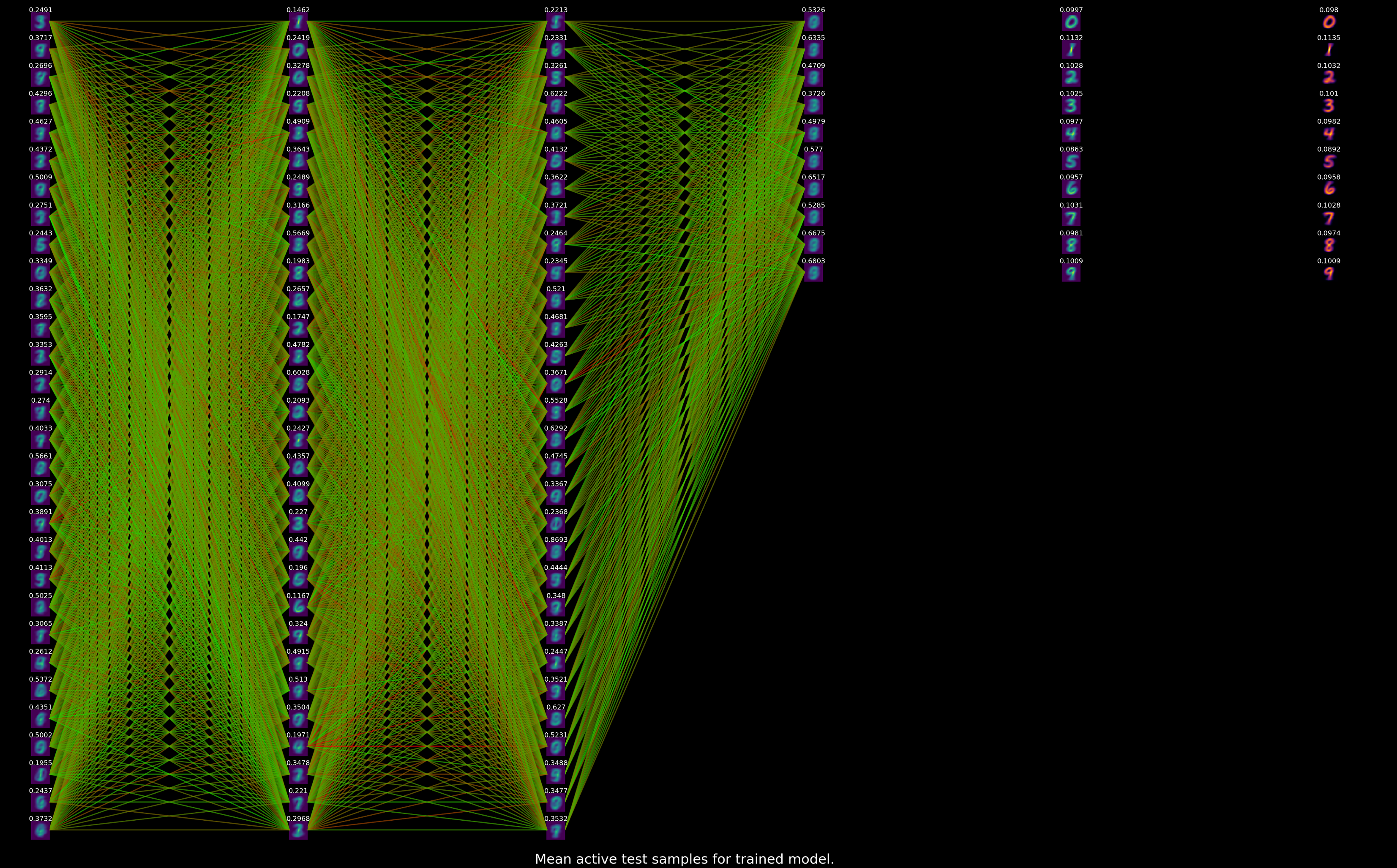