Postgraduate Studies in Computer & Electronic Engineering
The MUST research group offers a postgraduate programme focused on machine learning. To qualify, prospective students must have an undergraduate degree in a related Engineering field and an interest in machine learning, deep neural networks, statistical pattern recognition and/or generalisation within the context of Artificial Intelligence.
MUST students either study the essence of the learning process of different types of deep networks, or apply and improve these techniques in the context of a specific application domain. In practice, students work with MUST researchers on one of our research projects, using popular deep learning tools (such as Pytorch and Tensorflow) to explore specific questions on new and existing data sets. Our students learn about machine learning algorithms, development of software, and the design and interpretation of machine learning experiments. In addition to the generic skills, many of our students working on specific application domains gain the knowledge and skills required to effectively operate in these specialised domains.
Through its affiliations and collaborative activities with SANSA, CAIR and NITheCS, MUST is able to offer a number of M.Eng and PhD bursaries annually. We consider exceptional applications throughout the year (space allowing), and have an open application process in August-September.
MUST students enjoy considerable in-group support during their studies. Senior students are available to assist new students, resources are regularly shared on the group’s various communication channels, students meet with the entire group weekly and at least every second week with their supervisors in smaller groups. There's even a dedicated socials organiser to ensure an appropriate portion of fun and downtime.
Besides learning how to conduct research, postgraduate study at MUST is also a “finishing school” of sorts for engineers. Our students work in an informal office setting where they learn to balance the freedom to explore with a variety of responsibilities, such as contributing to group activities, participating in collaborative software development, sharing research results and guiding younger researchers when the time comes. Students leave MUST having learnt essential soft skills that will help them hit the ground running at their next opportunity, with a healthy understanding of work-life balance. Students at MUST also have the option of continuing their academic career, by applying to enrol for a PhD degree after completion of a master's degree.
We have concluded recruitment for postgraduate candidates in 2025. However, we are always open to considering exceptional students. Please feel free to reach out if you would like to be considered.
Recent topics
- Deep learning theory: Generalisation
- Activation functions in deep neural networks (MEng, 2019)
- Parametric studies of translation invariance and distortion robustness in Convolutional Neural Networks (MEng, 2020)
- Contrasting Convolutional Neural Networks with alternative architectures for transformation invariance (MEng, 2020)
- Generalisation in deep learning: Bilateral synergies in MLP learning (PhD, 2021)
- Exploring data utilisation in neural networks (MEng, 2021).
- Margin-based generalisation prediction in deep neural networks (PhD, 2024)
- Exploring Generalisation in deep convolutional neural networks using information flow (PhD, current)
- Margin-based regularization for deep neural networks (MEng, current)
- Exploring the effect of clustered hidden representations on generalisation in DNNs (MEng, current)
- Deep learning theory: Interpretability
- Interpreting deep neural networks with sample sets (MEng, 2022)
- Evaluating attribution for multivariate time series (MEng, current)
- Visualising feature effects for deep time series models (MEng, current)
- Interpreting spatio-temporal models (MEng, current)
- Space weather applications of DNNs
- Deep neural networks for prediction of solar flares (MEng, 2020)
- Interpretability of deep neural networks for SYM-H prediction (MEng, 2020)
- Knowledge discovery with additive attribution methods for geomagnetic index prediction (MEng, current)
- Speech and language applications of DNNs
- Embedding recognised speech in a multilingual environment (MEng, 2020)
- Classifying recognised speech with deep neural networks (MEng, 2020)
- Domain adaptation for speaker diarisation in low-resource environments (MEng, 2021)
- Automatic speech recognition on poor quality audio using Generative Adversarial Networks (MEng, 2021)
- Whale call detection with deep neural networks (MEng, 2023)
- Other applications of DNNs
- Traffic flow prediction with graph convolutional networks in under-resourced environments (MEng,2022)
- Channel estimation and equalisation using generative adversarial networks (MEng, 2022)
- Exploring tabular transformers for short-term insurance modelling (MEng, 2024)
-
Adaptive channel estimation using deep learning for future networks (PhD, current)
Study leaders
Prof Marelie Davel, Prof Stefan Lotz, Prof Samuel Tsoeu, Prof Etienne Barnard, Dr Tian Theunissen, Dr Randle Rabe and Dr Emmanuel Tuyishimire.
Domain experts participate as co-supervisors where relevant. Currently, the group collaborates with the following experts:
- Prof Albert Helberg, Electronic, Electrical and Computer Engineering, NWU & Telenet.
- Prof Rojanette Coetzee, Industrial Engineering, NWU
- Mr Ian Thomson, Electronic, Electrical and Computer Engineering, NWU & Beyond Limits
- Dr Johan Bosman, Mechanical Engineering, NWU & Jonker Sailplanes.
- Dr Charl van Heerden, SAIGEN.
Image gallery
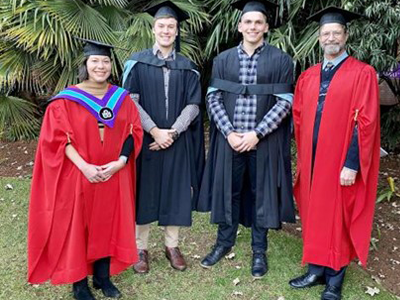
MEng Graduation (2023)
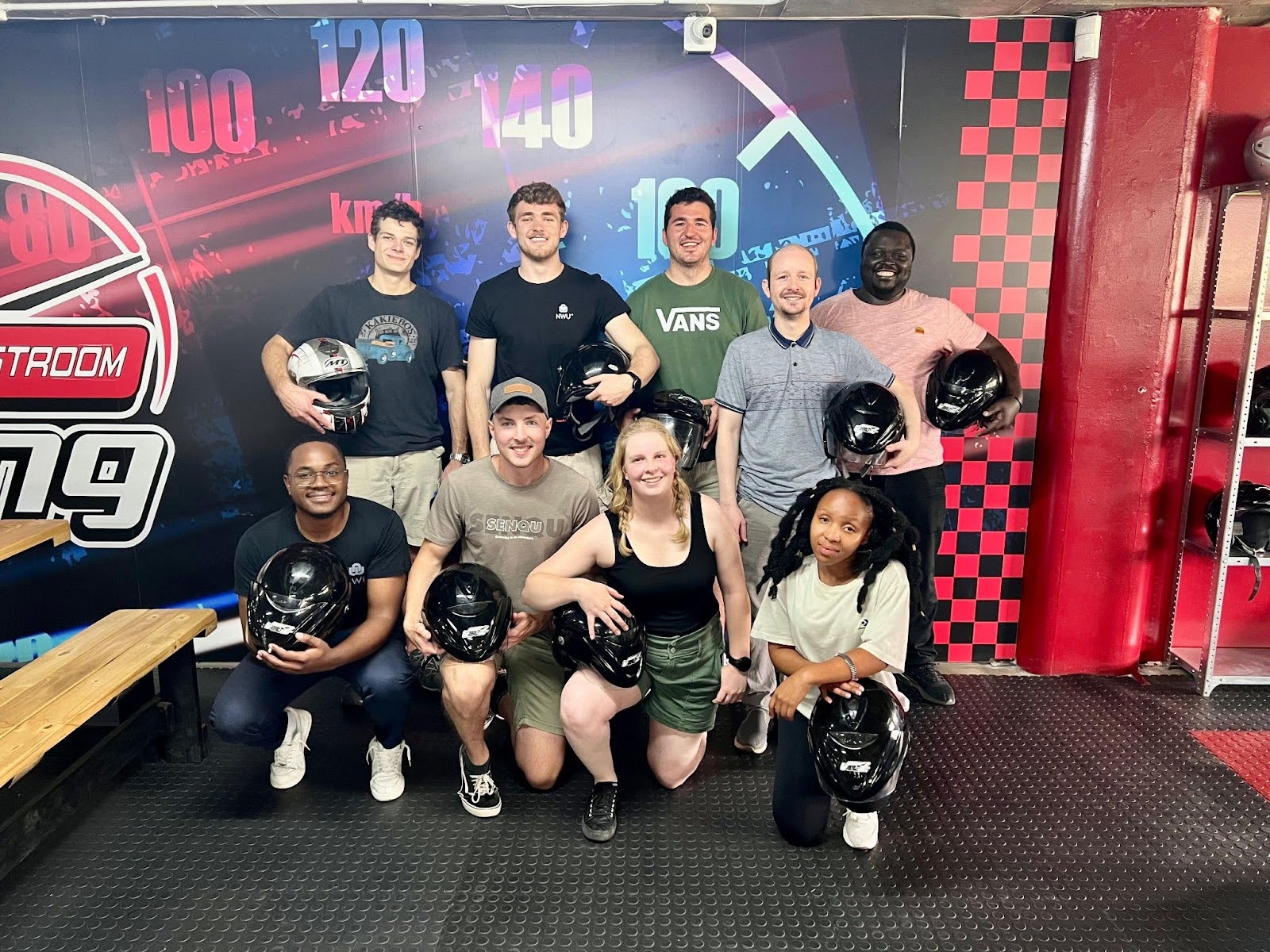
Potchefstroom year-end social event (2024)
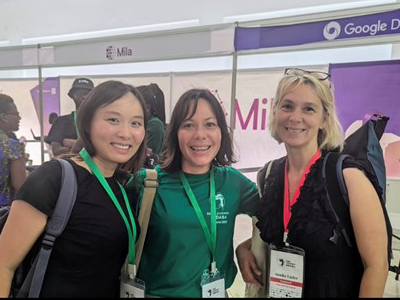
Deep Learning Indaba, Ghana (2023)
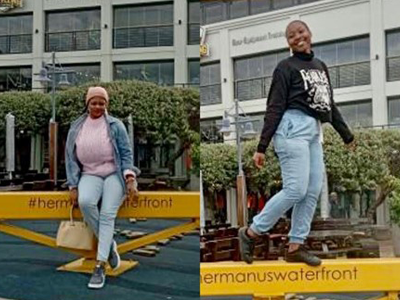
Study visit Hermanus (2023)
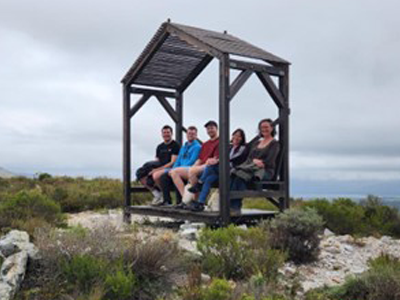
Study visit Hermanus (2023)
R.jpg)
Picnic, study visit in Hermanus (2022)
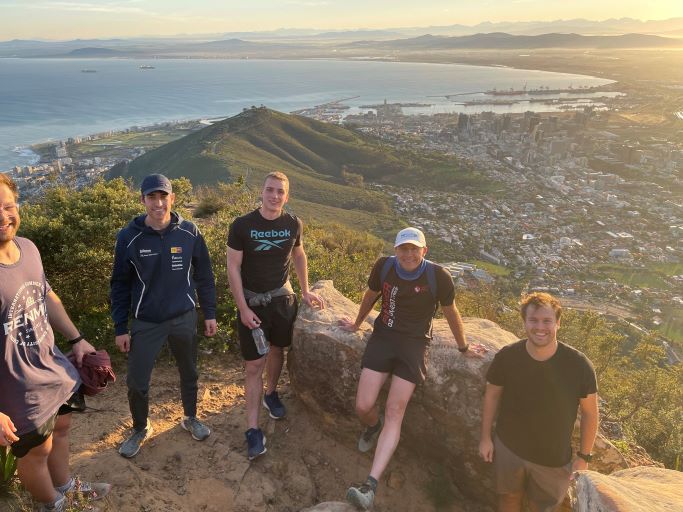
Student visit to SAIGEN (2021)
R.jpg)
In-person graduations return (2022)
R.jpg)
Deep Learning Indaba, Tunisia, (2022)
Sundowners at Fick's Pool (2021)
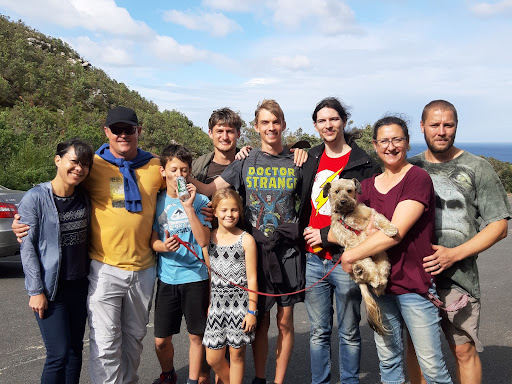
Fernkloof hike, study visit in Hermanus (2019)
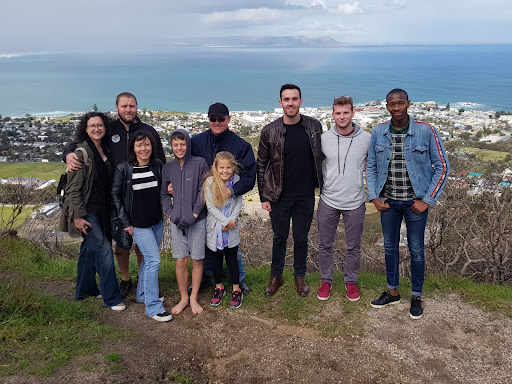
Scenic drive up Rotary Way, study visit in Hermanus (2019)
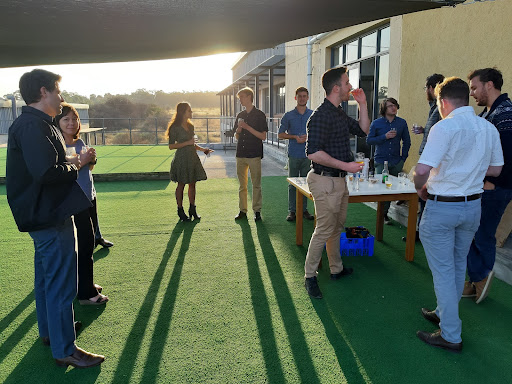
Post-colloquium beer tasting to celebrate 7 title registrations (2019)
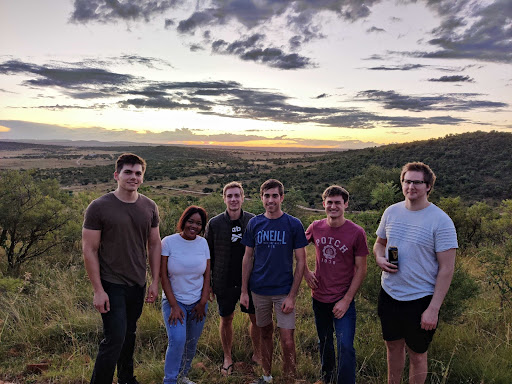
Potchefstroom social event (2021)
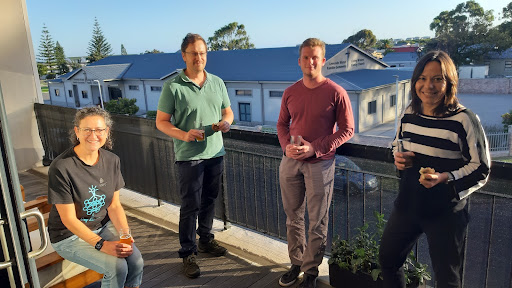
On the balcony of the new Hermanus office (2021)